(adj.) worthy of attention; remarkable
"We back notable founders pursuing world-changing ideas."
We can’t wait to say
“We knew you when.”
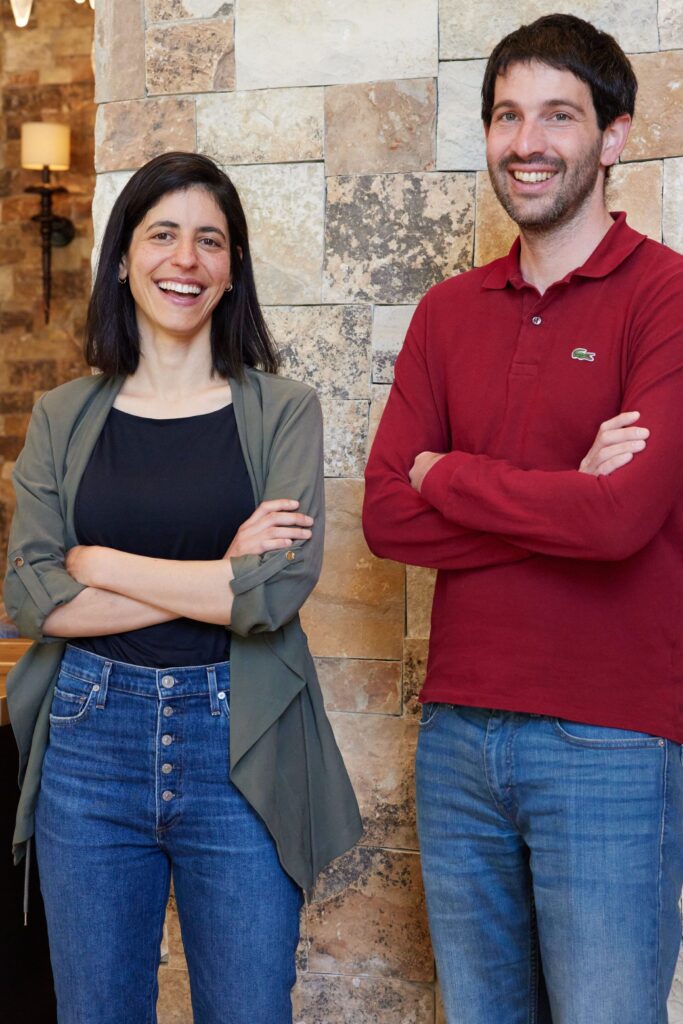
We're here for your journey.
Building a company is hard. Whether you are trying to build the best executive team, fundraise, refine your ICP, tell your story, or increase engineering velocity—we're here to support you.
We know how much the people equation matters, which is why we've created a learning platform, Founders + Leaders, to give you and your team access to expert leadership workshops, content, and community.
Pictured: Barr Moses & Lior Gavish, Monte Carlo Co-founders
We've leveraged our global perspective throughout our 20-plus year history to invest in the best across more than 200 companies, 30 IPOs, and $4.2B AUM.
13
Like you, we are focused on the long term. Strong, enduring relationships are built over time. The partners of Notable Capital have worked together for an average of 13 years.
70%+
Founder and LP relationships are our firm's lifeblood. More than 70% of our new investments are referred by a founder we've backed or have a portfolio founder as a co-investor.
1,000+
Founders connect with more than 1,000 other executives in our Founders + Leaders community, which includes cohorts and leadership training.